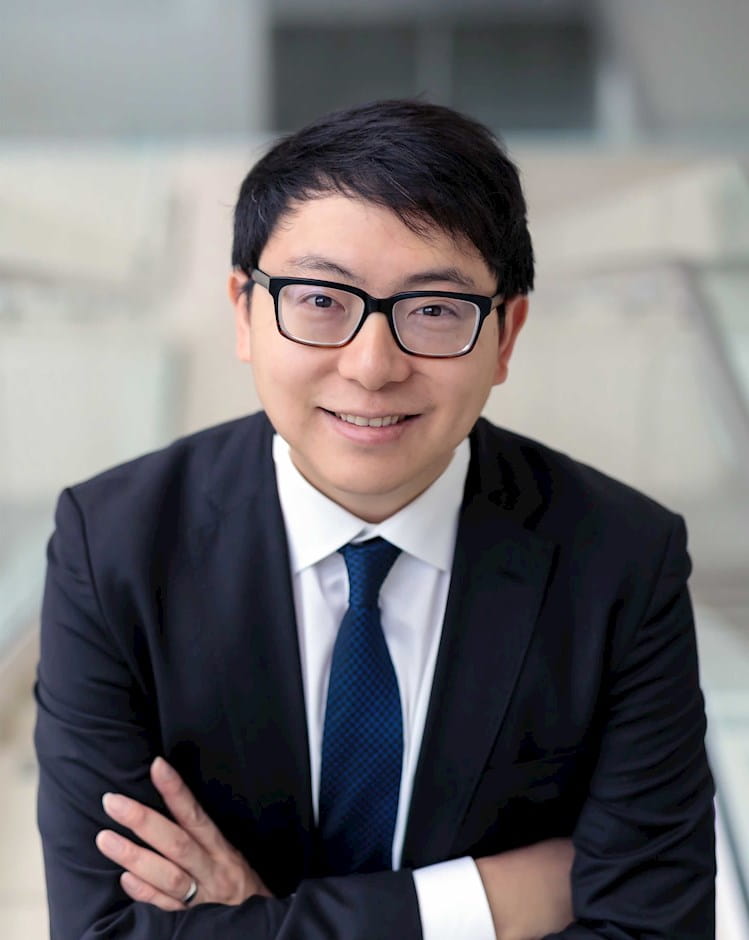
Dacheng Xiu
Joseph Sondheimer Professor of Econometrics and Statistics
Joseph Sondheimer Professor of Econometrics and Statistics
Dacheng Xiu’s research interests include developing statistical methodologies and applying them to financial data, while exploring their economic implications. His earlier research involved risk measurement and portfolio management with high-frequency data and econometric modeling of derivatives. His current work focuses on developing machine learning solutions to big-data problems in empirical asset pricing.
Xiu’s work has appeared in Econometrica, Journal of Political Economy, Journal of Finance, Review of Financial Studies, Journal of the American Statistical Association, and Annals of Statistics. He is a Co-Editor for the Journal of Financial Econometrics, an Associate Editor for the Review of Financial Studies, Management Science, Journal of Econometrics, Journal of Business & Economic Statistics, Journal of Applied Econometrics, the Econometrics Journal, and Journal of Empirical Finance. He has received several recognitions for his research, including the Fellow of the Society for Financial Econometrics, the Fellow of the Journal of Econometrics, the 2018 Swiss Finance Institute Outstanding Paper Award, the 2018 AQR Insight Award, and the Best Conference Paper Prize at the 2017 Annual Meeting of the European Finance Association.
In 2017, Xiu launched a website that provides up-to-date realized volatilities of individual stocks, as well as equity, currency, and commodity futures. These daily volatilities are calculated from the intraday transactions and the methodologies are based on his research of high-frequency data.
Xiu earned his PhD and MA in applied mathematics from Princeton University, where he was also a student at the Bendheim Center for Finance. Prior to his graduate studies, he obtained a BS in mathematics from the University of Science and Technology of China.
Why some machine learning models unlock economic forecasting potential.
{PubDate}Ten ways investors are, or should be, using large language models
{PubDate}The revolution that turned words into analyzable data continues to progress.
{PubDate}